Abstract
Recently, stereo vision based on lightweight RGBD cameras has been widely used in various fields. However, limited by the imaging principles, the commonly used RGB-D cameras based on TOF, structured light, or binocular vision acquire some invalid data inevitably, such as weak reflection, boundary shadows, and artifacts, which may bring adverse impacts to the follow-up work.
In this paper, we propose a new model for depth image completion based on the Attention Guided Gated-convolutional Network (AGG-Net), through which more accurate and reliable depth images can be obtained from the raw depth maps and the corresponding RGB images. Our model employs a UNet-like architecture which consists of two parallel branches of depth and color features. In the encoding stage, an Attention Guided Gated-Convolution (AG-GConv) module is proposed to realize the fusion of depth and color features at different scales, which can effectively reduce the negative impacts of invalid depth data on the reconstruction. In the decoding stage, an Attention Guided Skip Connection (AG-SC) module is presented to avoid introducing too many depth-irrelevant features to the reconstruction. The experimental results demonstrate that our method outperforms the state-of-the-art methods on the popular benchmarks NYU-Depth V2, DIML, and SUN RGB-D.
Method
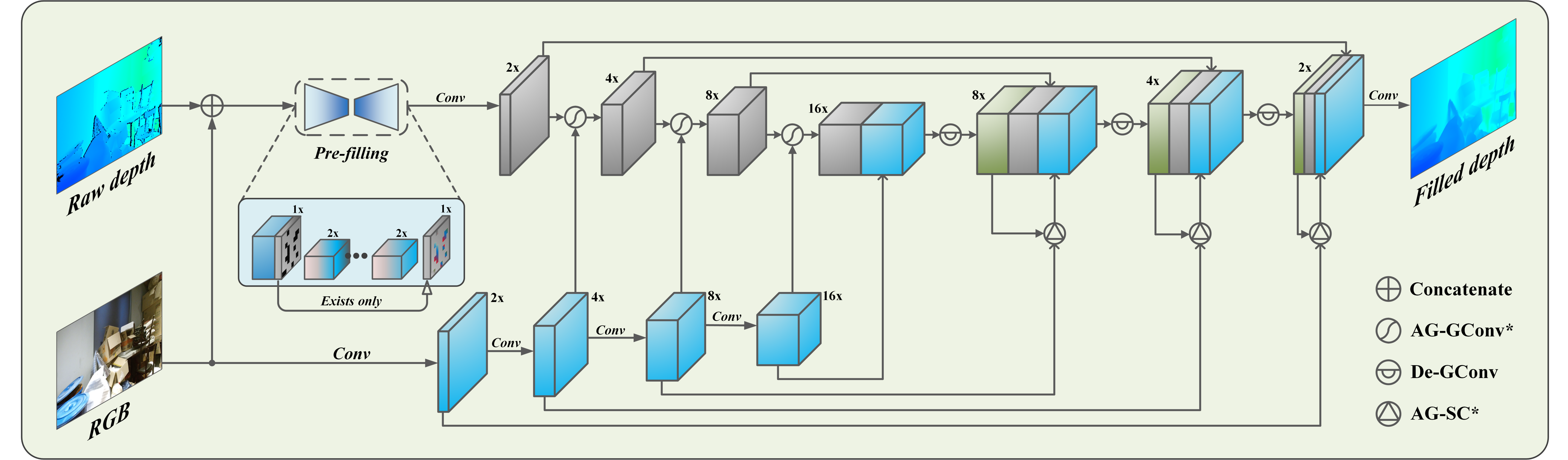
The pipeline of our model comprises two successive networks: the pre-filling network and the fine-tuning network. The former takes the raw depth images with the missing area and the corresponding RGB images as inputs and provides a complete depth map by filling all the missing values coarsely through a lightweight autoencoder. The fine-tuning network employs a dual-branch encoder to extract features from both depth and color images. Then it reconstructs the depth images through a multi-scale skip-connected decoder. Furthermore, the proposed AG-GConv and the AG-SC modules are embedded into the encoder and decoder layers, respectively, strengthening the fusion of the two modalities more reasonably and consequently improving the quality of the reconstructed depth images. The whole pipeline will be trained in an end-to-end fashion.
Results
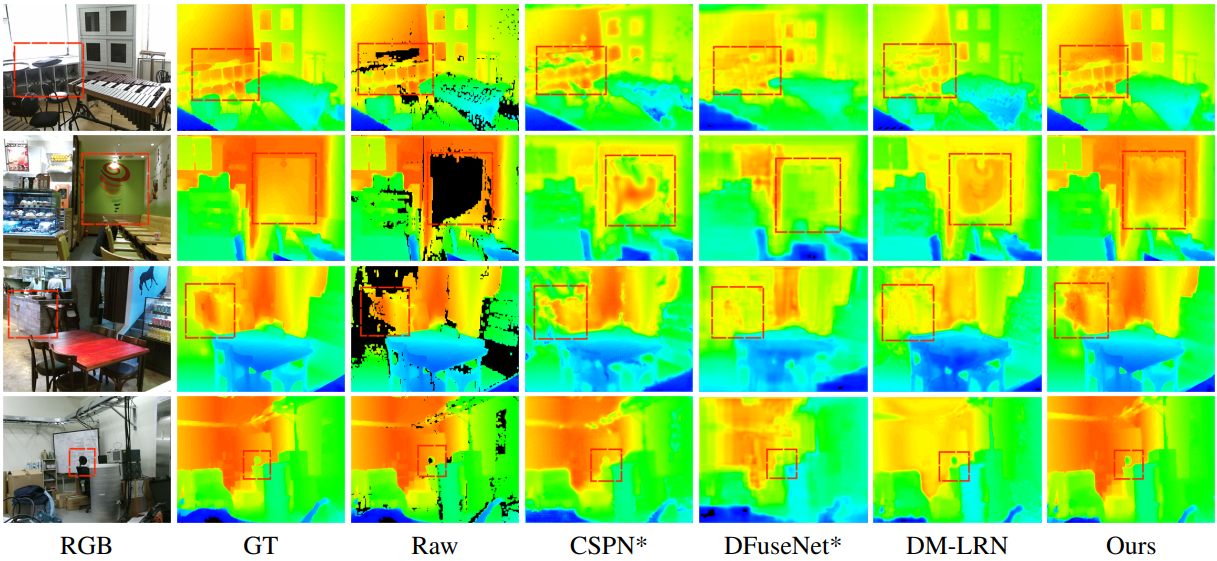
BibTeX
@inproceedings{chen2023agg,
title = {AGG-Net: Attention Guided Gated-convolutional Network for Depth Image Completion}
author = {Chen, Dongyue and Huang, Tingxuan and Song, Zhimin and Deng, Shizhuo and Jia, Tong},
title = {Nerfies: Deformable Neural Radiance Fields},
booktitle = {ICCV},
year = {2023}
}